Conventional methods for block-based compressive sensing consider an equal number of samples for all blocks. However, the sparsity order of blocks in natural images could be different and, therefore, a various number of samples could be required for their reconstruction. In this paper, we propose an adaptive block-based compressive sensing scheme, which collects a different number of samples from each block. We show that by adapting the sampling rate, in addition to reducing the whole required number of measurements, the reconstruction performance would be improved, simultaneously. Simulation results verify the effectiveness of our proposed scheme, specially for multi-level pixel value images like Mondrian test image.
Publication:
-
S.H. Safavi , F. Torkamani-Azar, “Sparsity-Aware Adaptive Block-Based Compressive Sensing” , IET Signal Processing, vol. 11, no. 1, pp. 36-42, Feb., 2017. [PDF] [DOI] [Code]
Here you can find some results of this project:
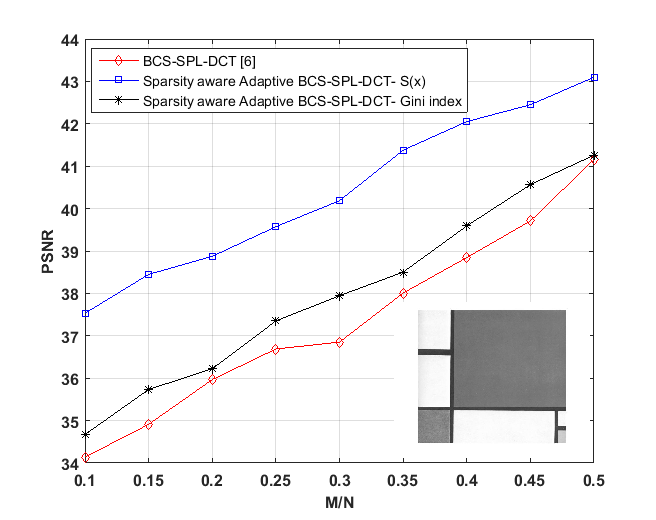
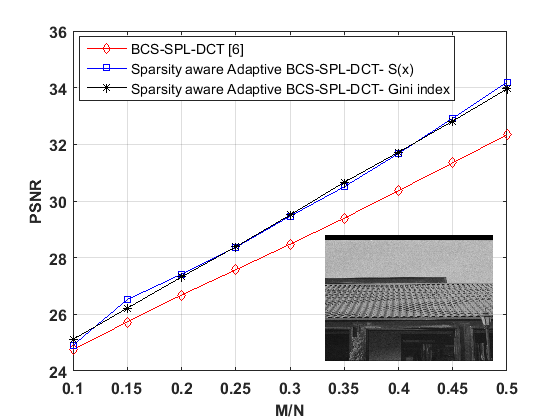
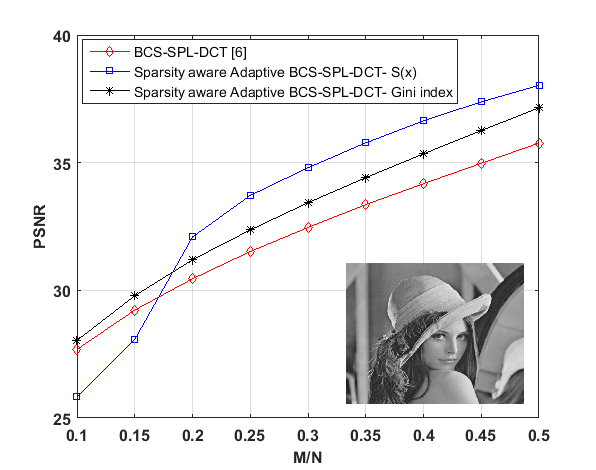
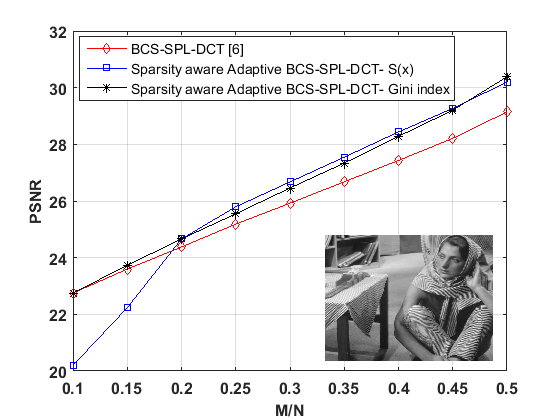